Dr. Yu from Purdue University visited Department of Civil Engineering
On December 13, 2018, Dr. Yu from Purdue University visited the Department of Civil Engineering, Tsinghua University, and gave a presentation titled "Deep Learning Accelerated Multiscale Failure Analysis of Composite Structures ". Professors Peng Feng and Wei Li of the Department of Civil Engineering of Tsinghua University and Professors Ren, Zhuang and Yao of School of Aerospace Engineering of Tsinghua University participated in this presentation and discussed the related contents with Dr. Yu.
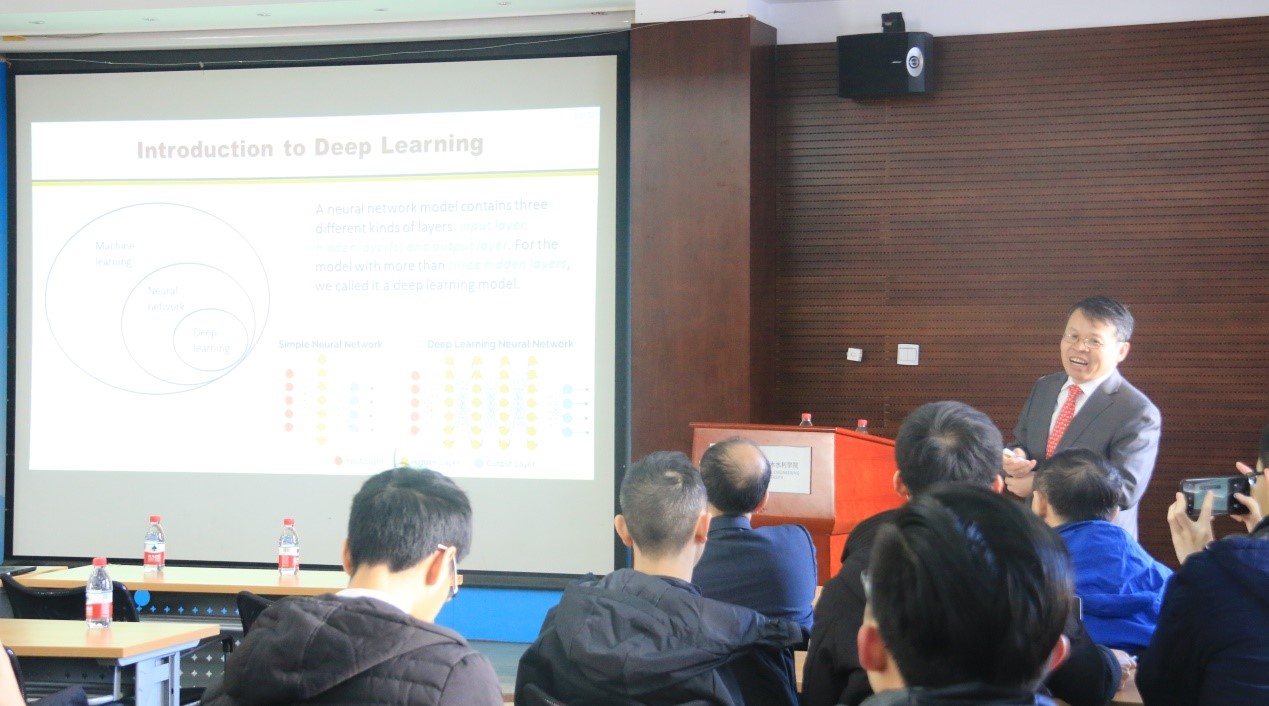
After almost five decades of research, failure and damage of composite structures remains unsolved. The main difficulties come from three inherent features of composites: anisotropy, heterogeneity, and multiscale architecture. Traditional approaches usually rely on assuming the basic composite building unit such as prepregs or woven fabrics as homogeneous materials to apply some failure initiation criterion. After the failure initiation criterion is meet, certain rules are used to degrade the material properties, and the composite structure is loaded until the structure cannot bear any more load. There are at least two issues associated with these approaches: 1) assuming the basic building unit as homogeneous is not valid because the stresses/strains used to evaluate failure are not real stresses/strains experienced either by any of the constituents; 2) the failure criterion and material degradation are assumed apriori, and do not have a rigorous thermodynamics foundation. This talk focuses on addressing the first issue through mechanics of structure genome (MSG), a recently developed revolutionary approach to multiscale modeling drastically different from the conventional bottom-up multiscale modeling approaches. MSG confines all approximations to the constitutive modeling for all types of structures including 3D solids, 2D plates/shells, and 1D beams. MSG allows one to choose the starting scale and ending scale and capture details as needed and affordable without invalid scale separation and assumptions within scales. Although MSG achieves the best compromise between efficiency and accuracy, it is not faster enough for failure and damage prediction. Deep learning is used to accelerate MSG-based failure analysis. The proposed method can be used to model any structures and materials featuring heterogeneity, anisotropy and multiscale architecture including but not limited to 3D printed materials, metamaterials, biomaterials, auxetic materials, smart materials, soft materials, etc.
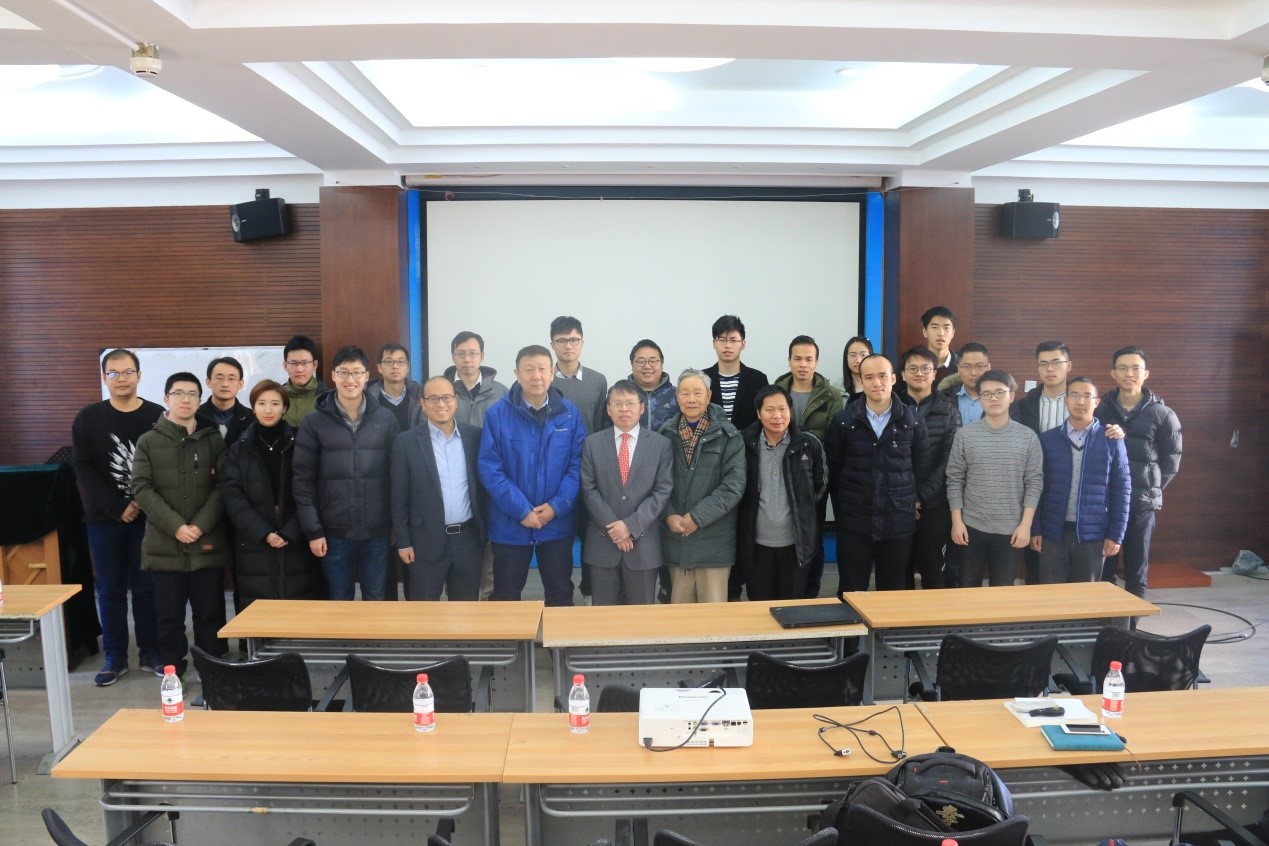
More ination about related research of Dr. Yu:https://cdmhub.org/groups/yugroup